
Intraspecific and interspecific interactions impact the extent and spatial patterning of animal home ranges (Adams 2001). detecting change in space use over successive years, evaluating the reliability of various home-range estimators). The use of intuitive and straightforward indices, like GOI, can be useful to promptly extract ecological information from such an amount of data (e.g. Modern biotelemetry offers huge amounts of data describing the space use of animal species. Within-colony overlap was elevated for both colonies (96.41% at Cassano delle Murge, n = 5 individuals 81.38% at Santeramo in Colle, n = 5 individuals), while between-colony overlap was low (19.12% n = 2 colonies) and, after a randomization procedure, more spatially-segregated than expected by chance. As a case study, we applied our index to 24,074 GPS points of 10 Lesser Kestrels ( Falco naumanni) in order to estimate within-colony and between-colony overlaps in two neighboring colonies in Southern Italy. Whatever the number of home ranges to be compared, GOI always returns a single score between 0 and 100. We propose here a new, conceptually simple and computationally efficient index (general overlap index GOI) for the ready computation within GIS of home range overlap of an arbitrarily large number (i.e., n ≥ 2) of individuals, populations or species. However, when the number of individuals, populations or species to be compared is elevated, an overlarge overlap matrix is difficult to interpret from an ecological viewpoint. So far, studies of home-range overlap typically make use of comparisons between pairs of individuals, populations or species, and return a matrix of pairwise overlaps (e.g., percent overlaps). Bayesian Belief Network analysis (BBN) was used to model the omnidirectional relationships of sleep duration and physical activity to history of stroke.There is increasing interest in evaluating home-range overlap (or, otherwise, segregation) between bird species, and between or within bird populations, to inform spatial planning. Method: Analysis was based on the 2004-2013 National Health Interview Survey (n=288,888). Such a model allowed us to develop a predictive classification model of stroke which can be used in post-stroke risk stratification and developing targeted stroke rehabilitation care based on an individual’s profile. The current study utilized Bayesian Belief Network analysis, a type of machine learning analysis, to develop profiles of physical activity (duration, intensity, and frequency) and sleep duration associated with or no history of stroke, given the influence of multiple stroke predictors and correlates. Though the interrelationships between PA, sleep, and other stroke risk factors have been studied, we are unclear about the associations of different types, frequency and duration of PA, sleep behavioral patterns (short, average and long sleep durations), within the context of stroke-related clinical, behavioral, and socio-demographic risk factors. Introduction: Physical activity (PA) and sleep are associated with cerebrovascular disease and events like stroke. Our work gives a theoretical explanation for the widespread empirical phenomenon of persistency for this LP relaxation. We design an algorithm to find stable blocks, and find that these real instances have large stable regions. We complement our theoretical results with an empirical evaluation of real-world MAP inference instances from computer vision. Under this block stability condition, we prove that the pairwise LP relaxation is persistent on the stable blocks. In this paper, we introduce a significantly more relaxed condition that only requires blocks (portions) of an input instance to be stable. Unfortunately, this strong condition does not seem to be satisfied in practice. The simplest stability condition assumes that the MAP solution does not change at all when some of the pairwise potentials are (adversarially) perturbed.

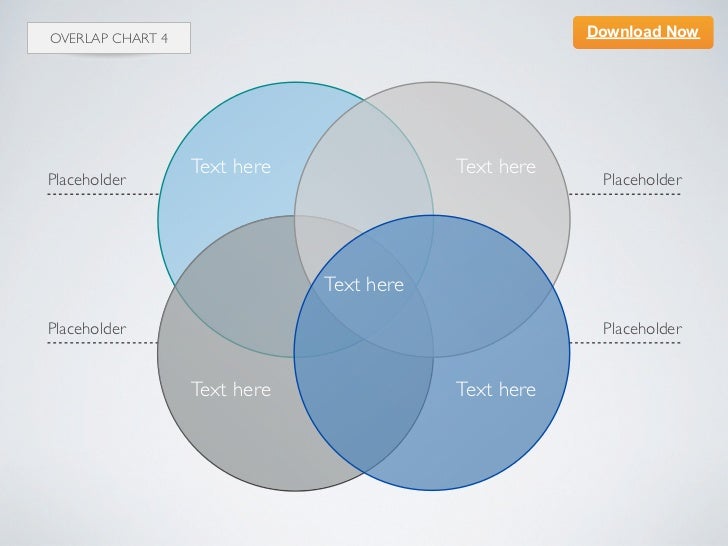
To understand the empirical success of approximate MAP inference, recent work (Lang et al., 2018) has shown that some popular approximation algorithms perform very well when the input instance is stable.
